Strategies for Mitigating Bias in AI
Bias is an inherent part of human behaviour, how do we navigate ethical challenges when training AI with models and data?
In the rapidly evolving landscape of artificial intelligence, ensuring ethical AI practices has become paramount for building trust and mitigating bias. As organizations increasingly rely on AI systems for decision-making processes, the risk of human bias infiltrating these technologies poses significant challenges. This document aims to unravel the complexities of AI bias, examining its origins from data and algorithmic sources, and highlighting the influence of human judgment. By exploring effective bias mitigation strategies, transparent development processes, and the implementation of ethical frameworks, we will provide a roadmap for businesses and public entities to navigate AI responsibly. Join us as we delve into the intricacies of ethical AI practices, offering insights to foster confidence and innovation within the industry.
Understanding AI Bias
Sources of AI Bias
Bias in AI systems often originates from two primary sources: data-driven biases and algorithmic biases. Data-driven biases occur when the historical data fed into AI models reflects existing prejudices or imbalances. For instance, if an AI system is trained on data that over-represents a particular demographic, its predictions or decisions may inherently favor that group, leading to skewed outcomes. Algorithmic biases emerge from the design and structure of the algorithms themselves. These biases can result from the assumptions made during the algorithm's creation, which might inadvertently favor certain outcomes over others. An example of this is when a machine learning model is programmed to prioritize efficiency over fairness, potentially leading to discriminatory results. Both types of biases underscore the importance of vigilance and thoughtful design in AI development to ensure ethical AI practices are upheld.
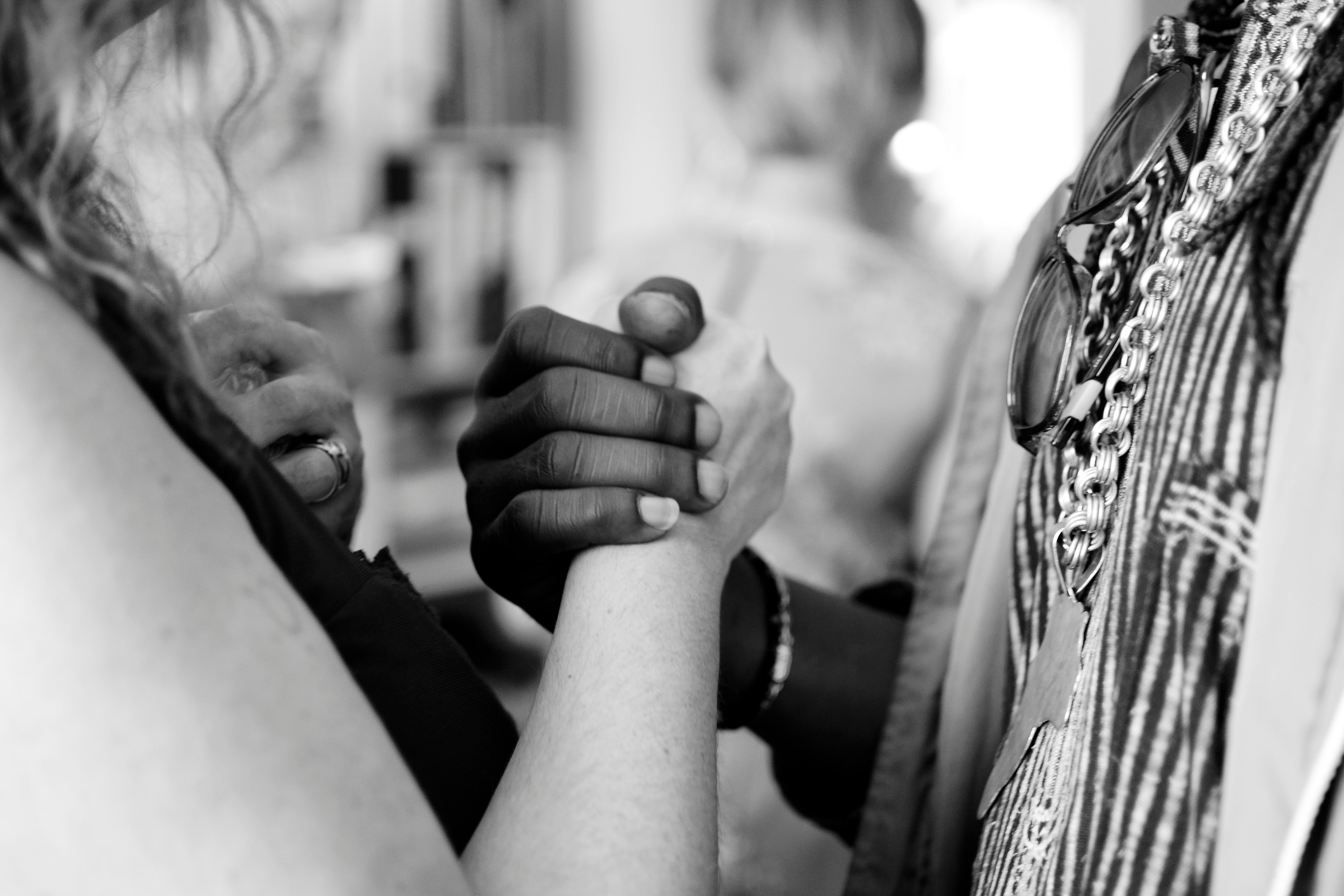
Human Contributions to AI Bias
Human influence plays a significant role in introducing bias within AI systems. The data used for training AI models is often curated and labeled by humans, making it susceptible to inherent biases. These biases may arise from the subjective perspectives or cultural backgrounds of the individuals involved in data preparation and labeling. Additionally, cognitive biases can infiltrate AI systems through programming. Developers may unknowingly embed their own biases into algorithms by choosing certain metrics or parameters that reflect their personal or organizational preferences. This can lead to AI systems that reflect and perpetuate human prejudices. Furthermore, the oversight and judgment exercised during AI development can be influenced by existing social biases, affecting how AI systems are deployed and evaluated. Addressing these human contributions requires a commitment to diverse and inclusive teams, along with rigorous scrutiny and testing of AI systems to mitigate bias and enhance fairness.
Impact of Bias on AI Decision Making
Real-world Implications
Bias in AI decision-making can have significant real-world implications, affecting both business outcomes and public sector operations. In business, biased AI systems might lead to unfair hiring practices, as seen in some recruitment software that disproportionately favors certain demographics over others. This not only undermines diversity and inclusion efforts but also limits the talent pool, ultimately impacting organizational performance. In the public sector, biased AI applications in areas such as law enforcement or social services can perpetuate systemic biases, resulting in unfair treatment or discrimination against minority groups. For instance, predictive policing algorithms have been criticized for disproportionately targeting communities of color, exacerbating existing social inequities. These examples highlight the critical need for bias mitigation strategies in AI development to ensure fair and equitable outcomes. Addressing AI bias is essential for maintaining public trust and achieving ethical AI practices that serve diverse populations effectively.
Mitigation Strategies
Mitigating bias in AI systems requires a multifaceted approach that combines technical solutions with organizational best practices. One effective strategy is implementing bias detection and correction techniques. These involve using statistical tools and machine learning models to identify and adjust for biases in datasets and algorithms. Another critical strategy is fostering transparency in AI development processes. By maintaining clear documentation and open communication about the decision-making logic and data used in AI systems, organizations can ensure accountability and facilitate external audits. Additionally, adopting ethical frameworks that prioritize fairness, accountability, and transparency is essential for guiding AI development and deployment. These frameworks should be integrated into organizational policies and practices to ensure consistent application across projects. Regular training and education for teams on AI ethics and bias awareness can further reinforce these efforts, equipping stakeholders with the knowledge to identify and address bias proactively. Together, these strategies can significantly reduce AI bias and enhance decision-making equity.
Ethics of AI and Responsible Use
Importance of Ethical Frameworks
Ethical frameworks in AI are crucial for ensuring that AI technologies are developed and used responsibly. These frameworks establish guidelines that prioritize values such as fairness, transparency, and accountability. By integrating these principles into AI systems, organizations can mitigate risks associated with AI bias and misuse. Ethical frameworks serve as a foundation for evaluating the impact of AI on society, helping to balance innovation with public welfare. They also promote trust between developers, users, and the communities affected by AI applications. For instance, implementing ethical standards can prevent discriminatory practices in areas like recruitment, lending, and law enforcement, where AI bias could have significant societal consequences. In the fast-paced world of technology, ethical frameworks provide a structured approach to navigating complex moral dilemmas, ensuring that AI systems contribute positively to society. Establishing and adhering to these frameworks is essential for responsible AI use and fostering confidence in AI technologies.
Responsibilities of Organizations
Organizations play a pivotal role in ensuring ethical AI practices are upheld throughout the lifecycle of AI systems. One key responsibility is the implementation of unbiased practices in AI development and deployment. This involves rigorous testing and validation processes to detect and rectify biases in algorithms and datasets. Moreover, organizations should establish clear ethical guidelines and policies that govern AI use, ensuring compliance with legal standards and societal expectations. Educating teams on AI ethics is another critical responsibility. Providing training and resources enables employees to recognize potential biases and ethical dilemmas, fostering a culture of accountability and awareness. Organizations should also engage with diverse stakeholders, including ethicists and community representatives, to gain insights into the societal impact of their AI initiatives. By prioritizing these responsibilities, organizations can not only mitigate the risks associated with biased AI but also enhance trust and confidence in their AI solutions, driving sustainable innovation.